Melia: An Expressive Harmonizer at the Limits of AI
Matthew Caren; Joshua Bennett
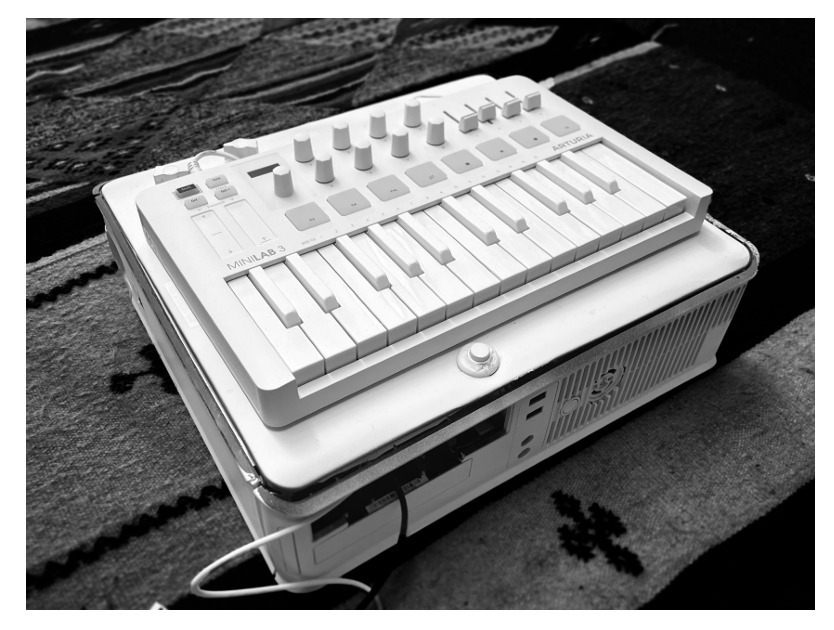
- Format: poster
- Session: posters-3
- Paper PDF link
- Presence: remote
- Duration: 5
- Type: short
Abstract:
We present Melia, a harmonizer-like digital instrument that explores how common failure modes of machine learning and artificial intelligence (ML/AI) systems can be used in expressive and musical ways. The instrument is anchored by an audio-to-audio neural network trained on a hand-curated dataset to perform pitch-shifting and dynamic filtering. Biased training data and poor out-of-distribution generalization are deliberately leveraged as musical devices and sources of instrument-defining idiosyncrasies. Melia features a custom hardware interface with a MIDI keyboard that polyphonically allocates instances of the model to harmonize live audio input, and integrated hardware controls that manipulate model parameters and various audio effects in real-time. This paper presents an overview of related work, the instrument’s hardware and software, and how audio-to-audio AI models might fit into the long-standing tradition of musicians, artists, and instrument-makers finding inspiration in a medium’s shortcomings.