Introducing EG-IPT and ipt~: a novel electric guitar dataset and a new Max/MSP object for real-time classification of instrumental playing techniques
Marco Fiorini; Nicolas Brochec; Joakim Borg; Riccardo Pasini
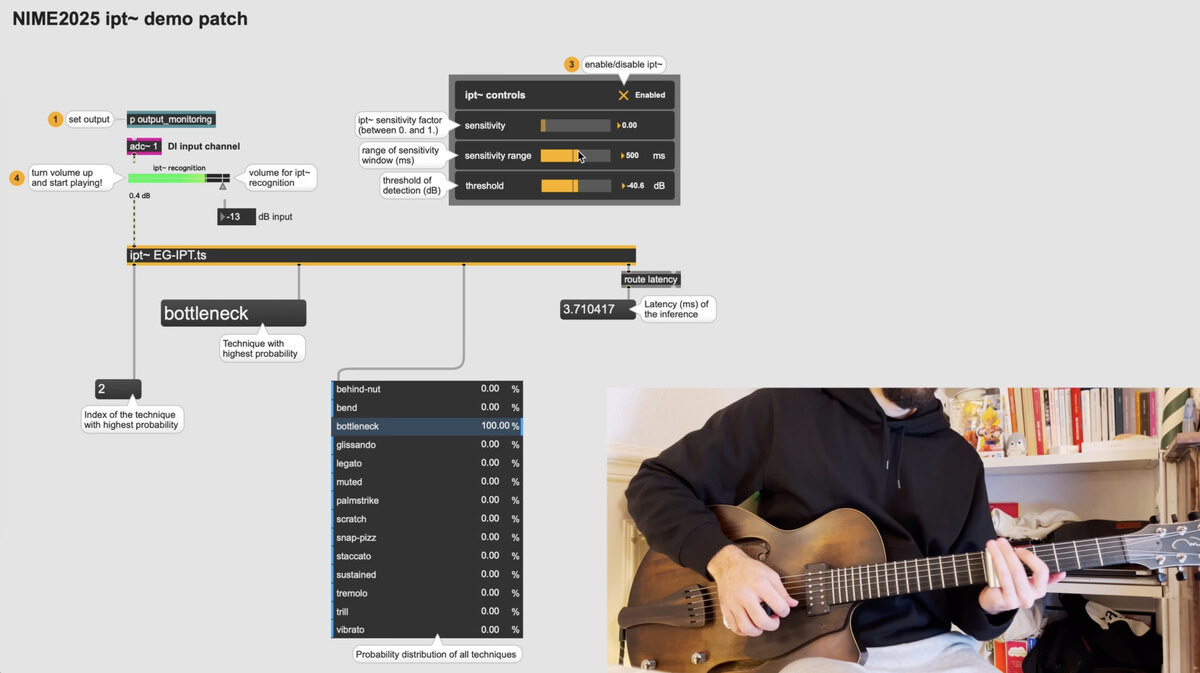
- Format: poster
- Session: posters-1
- Presence: in person
- Duration: 5
- Type: long
Abstract:
This paper presents two key contributions to the real-time classification of Instrumental Playing Techniques (IPTs) in the context of NIME and human-machine interactive systems: the EG-IPT dataset and the ipt~ Max/MSP object. The EG-IPT dataset, specifically designed for electric guitar, encompasses a broad range of IPTs captured across six distinct audio sources (five microphones and one direct input) and three pickup configurations. This diversity in recording conditions provides a robust foundation for training accurate models. We evaluate the dataset by employing a Convolutional Neural Network-based classifier (CNN), achieving state-of-the-art performance across a wide array of IPT classes, thereby validating the dataset’s efficacy. The ipt~ object is a new Max/MSP external enabling real-time classification of IPTs via pre-trained CNN models. While in this paper it’s demonstrated with the EG-IPT dataset, the ipt~ object is adaptable to models trained on various instruments. By integrating EG-IPT and ipt~, we introduce a novel, end-to-end workflow that spans from data collection, model training to real-time classification and human-computer interaction. This workflow exemplifies the entanglement of diverse components (data acquisition, machine learning, real-time processing, and interactive control) within a unified system, advancing the potential for dynamic, real-time music performance and human-computer interaction in the context of NIME.